On one hand, there are some who believe Long Covid afflicts 43% of Covid patients. On the other, some commentators say Long Covid is basically psychosomatic.
Neither extreme is correct.
And yet more than 3 years into the pandemic, the search for an objective medical finding that clinches a Long Covid diagnosis—be it blood markers, lung tests, specific cognitive tests, imaging—has come up short. As Derek Lowe wrote in a blog for the journal Science, “[S]o far there are no diagnostic findings that would allow you to even say for sure that post-Covid even exists, biochemically.”
Not everything has been ruled out. Nothing reliable has been ruled in. Right now, Long Covid diagnoses are made based on our best aggregations of commonly reported symptoms.
There’s no smoking gun. There’s no CT scan showing an infected colon (diverticulitis), or an ECG showing a blocked artery (heart attacks), or misshapen white blood cells collected from a bone marrow biopsy (leukemia). Diagnosing Long Covid comes down to patients filling out questionnaires.
New data goes beyond showing we can prevent Long Covid…
That’s why when I first saw the data of a blinded, randomized, placebo-controlled clinical trial (now published in the prestigious medical journal Lancet Infectious Diseases) showing that patients who had taken the diabetes drug metformin for two weeks subsequently had a 40% lower rate of Long Covid (6% down from 10%) than those who had received placebo, my eyes literally bugged out.
Was anyone else seeing what I was seeing? The researchers had not only shown that Long Covid might be preventable simply by taking a low-cost drug. They’d provided the best objective scientific proof that Long Covid measurably exists that I’d ever seen, or have seen since.
I called a few experts I trust, including some I know believe that Long Covid is both real and probably less common than reported in the medical literature. (I wouldn’t call them “skeptics,” because too often that term gets applied to deniers, which they aren’t.) My colleagues hadn’t seen the data, but they agreed with my take once we discussed it.
I found myself saying a phrase repeatedly. “If Long Covid were not real, or it were too inconsistently diagnosed, in any trial you conducted, your Kaplan-Meier curves would always be sad.”
Happy Kaplan-Meier curves.
What did I mean by that? Kaplan-Meier curves contain two lines. One line shows the rate of disease (or other outcome) in a group that received a drug being studied. The other shows the rate found in the control group. (Malcom Gladwell wrote a nice essay about this in The New Yorker back in 2010.) On the vertical Y-axis is the percent of patients with a certain outcome. On the horizontal X-axis is time. One curve shows the rates of symptoms, for example, in a study drug, while the other shows that for placebo. When the curves separate over time, that means the drug is working. Happy Kaplan-Meier. When the drug isn’t working, the curves never seperate. Sad Kaplan-Meieir. Take a look at an example:
In our imaginary study, by day 100, 19% of patients who took placebo had symptoms, while 15% of patients who took the study drug did. Was that difference meaningful? That depends on the size of the study, and other statistical tests. In this case, I left it intentionally ambiguous.
In some cases, though, the outcomes are really obvious, leading to dramatic Kaplan-Meier curves. One example: the results of the Covid-19 mRNA studies!
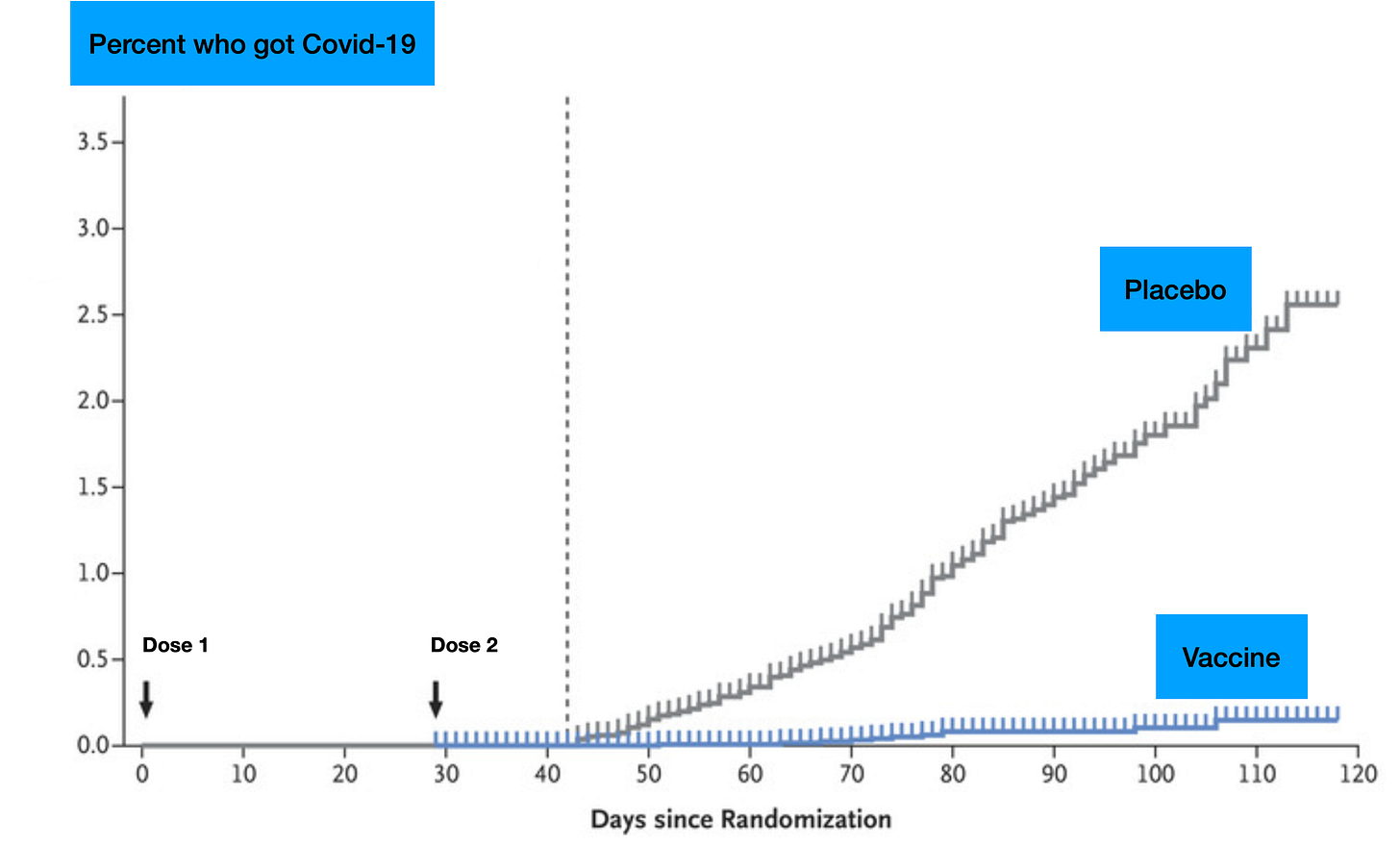
The statistical analyses bear out what your eye can plainly see on the Kaplan-Meier curve above. That’s what I call a happy Kaplan-Meier curve. The vaccines worked.
What if we were chasing a ghost?
On the other hand, what if we were chasing a mirage—something that looked real, but wasn’t. Think about it. If a disease either doesn’t truly exist, or does but is too poorly understood to distinguish it from other unrelated conditions, even the best researchers’ attempts to find a treatment would be doomed to fail. Again, again, and again.
Let’s do a thought experiment. Imagine there’s a news story of 100 people who have suddenly developed some new unknown medical condition that causes tingling of the hands. After the story airs, another 900 people report the symptoms. Researchers ask all 1,000 to join a trial in which the participants will either get a drug that might stop the tingling or a placebo, but they won’t know which they received (blinded).
Now, what if we know for a fact that the tingling is completely psychological; that is, it’s a “mass psychogenic illness” (or “mass hysteria,” a term which has fallen out of favor). Yes, the symptoms feel real, but they are not being caused by a medical illness or new exposure. No medical treatment could possibly decrease the rates of the tingling compared to the placebo. You could try hundreds of possible drugs. They’d all perform no better than placebo. Try as they might, researchers would keep hitting a brick wall.
That’s what I meant when I said if a disease were not real or too poorly understood “your Kaplan-Meier curves would always be sad.” No matter what treatment you tested, the lines on the Kaplan-Meier curves would never separate. The lines would be too close together. Sad!
Let’s picture 3 trials, each lasting 100 days. In each, volunteers are randomized to receive either a “study drug” or placebo and that in order for any differences to be statistically meaningful, the difference has to be 10% or greater.
All the study drugs failed. Sad Kaplan-Meier curves. There’s little-to-no daylight between those curves, let alone the 10% difference we specified would be a statistical success.
But now let’s imagine a different situation. This time, we are not sure if the condition is real (or sufficiently understood) and two study drugs are being tested versus placebo.
Study drug A is another disappointment. But, lo! Look Study drug B! Behold all that space between the drug and placebo groups by day 60, 70, 80, 90, and 100! This Kaplan-Meier curve is not sad. It is happy! The drug looks like it works. Patients randomized to received Study drug B had a 13% lower rate (15% versus 28%) of symptoms by day 100 (and a relative decrease of 46%). That’s more than the 10% threshold we had established. To me, these data would confirm two things. First, Study drug B worked. Second, it indicates that the definition of the condition being studied was good enough that the drug could modify the outcomes. In other words, enough of the participants had something similar enough that the same study drug helped them, as compared to placebo.
That is exactly what Dr. Carolyn Bramante and her colleagues found in their study measuring the rates of Long Covid in patients randomized to receive the drug metformin for two weeks instead of placebo during their period of initial infection.
Now that you know how to read a Kaplan-Meier curve, look at Bramante’s data (emoji added by me).
This impressed me. Those curves clearly separated a few months out, just when you’d start to see Long Covid diagnoses cropping up. Not only does the trial look successful, but (as I’ve been leading up to), it indicates to me that however imprecise Long Covid diagnoses may be, clinicians and researchers have done well enough at identifying patients that such a result was even possible! Remember, if a syndrome like Long Covid were too heterogenous, a treatment couldn’t change its rate of occurrence compared to placebo.
Having shared these thoughts with the journal editors at Lancet Infectious Diseases, I am proud that they invited me to write a commentary that was co-published alongside the trial. You can read that version of these thoughts here.
While I still believe we still need better definitions and diagnostics for Long Covid, the results of this trial tell me, for the first time, that we’re on the right track. This is cause for hope that we’ll be able to make progress in decreasing Long Covid incidence, and hopefully soon be able to treat cases with evidence-based therapies. Other studies are indeed underway. But today is a moment for optimism.
Great explanation regarding clinical trials and K-M curves as it applies to “syndromes” that we have not yet fully understood! Indeed it does appear that long COVID is real. The challenge now is to understand the pathobiology of the condition and today we can use ML to help us discover likely pathways. Thanks for a great write-up!
As a novice in pharmaceutical research, thank you for this awesome explanation on Kaplan-Meier curves!!